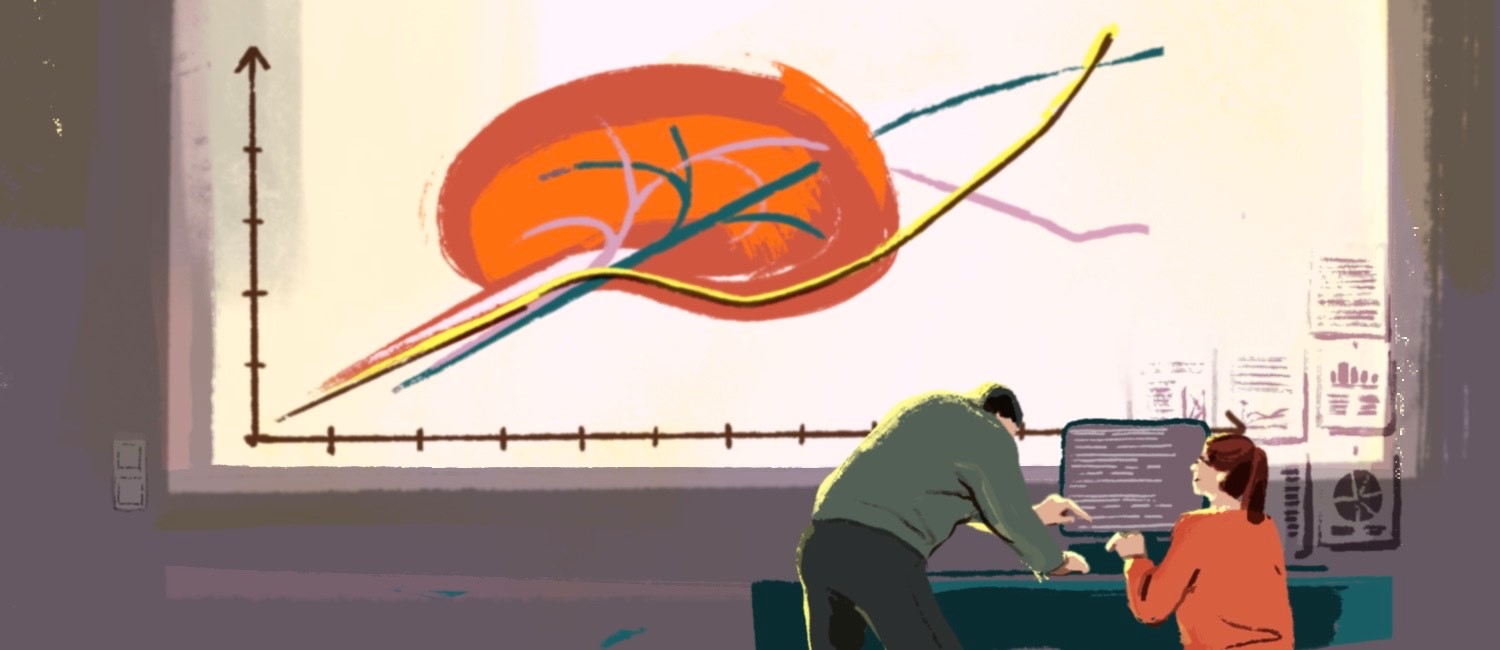
Based on the digital data from 270,000 US patients, last winter, eight data science students from the h_da launched an ambitious practical project aimed at generating prognostic models capable of predicting the outcome of specific kidney transplants. In other words, tools with which to calculate how successful any given transplant should be, currently a hot topic in view of long waiting lists and declining willingness to donate organs, whereby every single kidney becomes a vital asset. In terms of ring binders, this mass of data would fill an entire office floor.
By Christina Janssen, 30.9.2021
The semester project is titled ‘Data Mining Procedures for Organ Transplant Operations’. Not quite what anyone interested in a Data Science degree course, by dint of their current image in the media as career-enhancing and thus ‘sexy’, would expect. Or might this be wrong? Julia Psenner, Franziska Schmidt and Roman Keßler took part in the project and found it fascinating: “although such a heterogeneous group of mathematicians, computer scientists, a physicist and a neuro-scientist, we all worked so well together and learnt so much from each-other”.
From a mountain of data
It all began with a veritable mountain of data, which supervising lecturers mathematics professor Antje Jahn and computer science professor Gunter Grieser, simply presented to their student team, according to the motto – now do something useful with it. Specialist for theoretical computer science and artificial intelligence Grieser grins “there’s order behind chaos”. “We try to give our students plenty of room to develop their own solutions, instead of tying them to fragmented assignments.”
![[Translate to Englisch:] Portraitbilder h_da-Studierende Julia Psenner, Roman Kessler und Franziska Schmidt](/fileadmin/Bilder/Meldungen/Das_Chaos/21_09_29_Collage_Studis.jpg)
The team employed a scrum-approach: they met every three weeks and chose assignments out of the large pool of necessary tasks, which then had to be completed by the next meeting. As Roman Keßler points out “initially of course, we needed to form an overview of the data involved – which proved to be more difficult than expected”. Not surprising, as each ‘patient’s file’ consisted of a table with hundreds of columns, such as age, gender, height, weight, blood group, smoker or non-smoker, medical history, how the operation went, survival time, rejection reactions, along with numerous immunological parameters.
Franziska Schmidt says “first we needed to dig in and find out what all of the many medical abbreviations and variables meant”. The data was sometimes even contradictory or incomplete. “The mathematical models cannot deal with gaps in the data, so in such cases we had to figure out and insert estimates.” Yet as Franziska Schmidt explains, precisely these aspects are one of the data science degree course’s strengths. She points out that “each project requires us to figure out how to deal with a new field or area, so whilst we didn’t become medical experts through working out organ transplant data, we nonetheless acquired a large amount of ‘domain insights’” Whilst for some this is demanding, others find it stimulating.
After an initial, basic pre-selection – children, 60 years plus and living donations were all sifted out – roughly 70,000 data sets remained for the students to process. They developed a total of five prognostic models with names that are cryptic for laypersons, but which are bandied around with ease by the young students. How about the ‘Cox-Regressionsmodel’, the ‘Accelerated Failure Time-Model’ or perhaps you’d prefer the ‘Random Survival Forest’? Two of the processes are based on classic statistical models, the other three on machine learning. Professor Antje Jahn describes the unexpected results thus: “basically, the prognostic capacities of all the models is equally good”.
Glass box versus black box
Fundamental differences remain. Bio-statistician Jahn explains that “the model-based methods require less processing power, produce good results and are moreover easier to explain – something close to our hearts in this project. This means it’s easier to understand how the results are generated, quite different to the ‘more modern’ machine learning methods. For although these produce a prognosis for every patient, it’s often not so easy to explain why the result is so precise.” Machine learning processes are also often akin to forests full of trees and leaves, adds Grieser: “If a leaf sways in the wind this will affect neighbouring leaves, and so on.” He therefore describes the model-based, statistical methods as a glass box which one could see into. By contrast, machine learning models are often a black box. This difference could play a relevant role in future applications.
A further important insight for the students is that the choice of statistical method is less decisive for the accuracy of a prognosis than is rigorous processing of the data sets. Franziska Schmidt reckons “we learned that as a rule of thumb, 80 per cent of the time available should be used to prepare the data and only the remaining 20 per cent to working on the models themselves”. The students used the programming language ‘R’. Julia Psenner, who worked on the inter-disciplinary project, describes how they collaborated “we learned a lot from a computer scientist on our team who had specialised in the language. He always delivered and was great at explaining things. Although at first this made me feel slightly insecure, I soon discovered that I was also able to utilise and integrate my own mathematical competencies.” The dream is of combining interdisciplinary skills to streamline projects: planning together, learning from and with one-another and at the end of the day successfully completing a project.
Up until recently there was no organ transplant registry in Germany
Jahn and Grieser are likewise pleased with the results. The models were never intended to be put to practical use, but this is only the norm and to be expected of study projects. However, within six months of intensive work, the future data scientists have laid the foundations for future research projects. In June 2021 an organ transplant registry was finally established in Germany. Jahn and Grieser have been waiting a long time for this to happen. Now they aim to use German data as well, in order to glean fresh insights – in collaboration with the ‘Deutschen Stiftung Organspende’.
For anyone concerned about data protection: the anonymous data processed by the h_da students comes from the US Transplant Registry, from 1980 to the current day. The data was voluntarily made available for research purposes by the patients themselves. Data pertaining to both donors and patients will likewise be processed at the Deutschen Transplantationsregister so that no persons can be identified. This enables Grieser and Jahn – possibly as one of the first research teams in Germany – to be rigorous in evaluating the data. As Grieser points out “what really interests us is whether the data can be used to determine new links between individual parameters and the actual success of a transplant operation. Connections as yet unknown to medical science”. If this is the case, the next step could be to create a prototype prognostic tool which utilises models similar to those created by the students. “This is of course only feasible in cooperation with partners from the medical field.”
Such a tool as envisaged by Jahn and Grieser would be intended to assist both doctors and patients. “For instance, if a patient requires a new kidney and then needs to decide whether to accept the first offer, or wait for the organ of a donor who never suffered from any serious infections, or only donors aged under 20.” The tool could then be used to select specific criteria and observe how these affect the prognoses. During the practical project one of the students envisaged developments which Jahn and Grieser highly praise. “I can see an app with virtual slide controls enabling me to enter in, for example: I’m 50 old, non-smoker, I weigh 70 kilos, have been on dialysis machines for the past 17 years and so on. This would enable patients to try out different scenarios, according to the data entered.”
Splendid job opportunities
Whilst still a vision, the students have certainly contributed to such models one day becoming reality. Julia Psenner is currently writing her thesis whilst working for a company, Roman Keßler is writing his from a university in Norway, and simultaneously working on his dissertation in neuro-science. Franziska Schmidt remains at the h_da and is preparing the next steps of the transplant project: insights gleaned during the practical project are planned to be transferred to the German data as soon as possible. A funding request has been made to the Federal Ministry for Education and Research, and now team Jahn – Grieser await the verdict.
One thing is certain: the youthful data scientists don’t need to be concerned about their choice of jobs in the future. Because for graduates of the masters degree course in Data Science, established five years ago by the h_da as the first German university to do so, every door in the job market will be wide open.
Translation: Paul Comley
Contact details
Christina Janssen
Scientific editor
Tel.: +49.6151.16-30112
E-Mail: christina.janssen@h-da.de
Interdisciplinary Team
Mathematics Prof. Dr. Antje Jahn and computer scientist Prof. Dr. Gunter Grieser have laid down a challenge for the prospective data scientists. So far as feasible, they are to utilise data from US transplant patients in order to develop prognostic models. Jahn supervises the study course Data Science at the h_da together with a colleague from the Computer Science faculty. At the Computer Science faculty, Grieser is a professor of theoretical computer science and artificial intelligence.